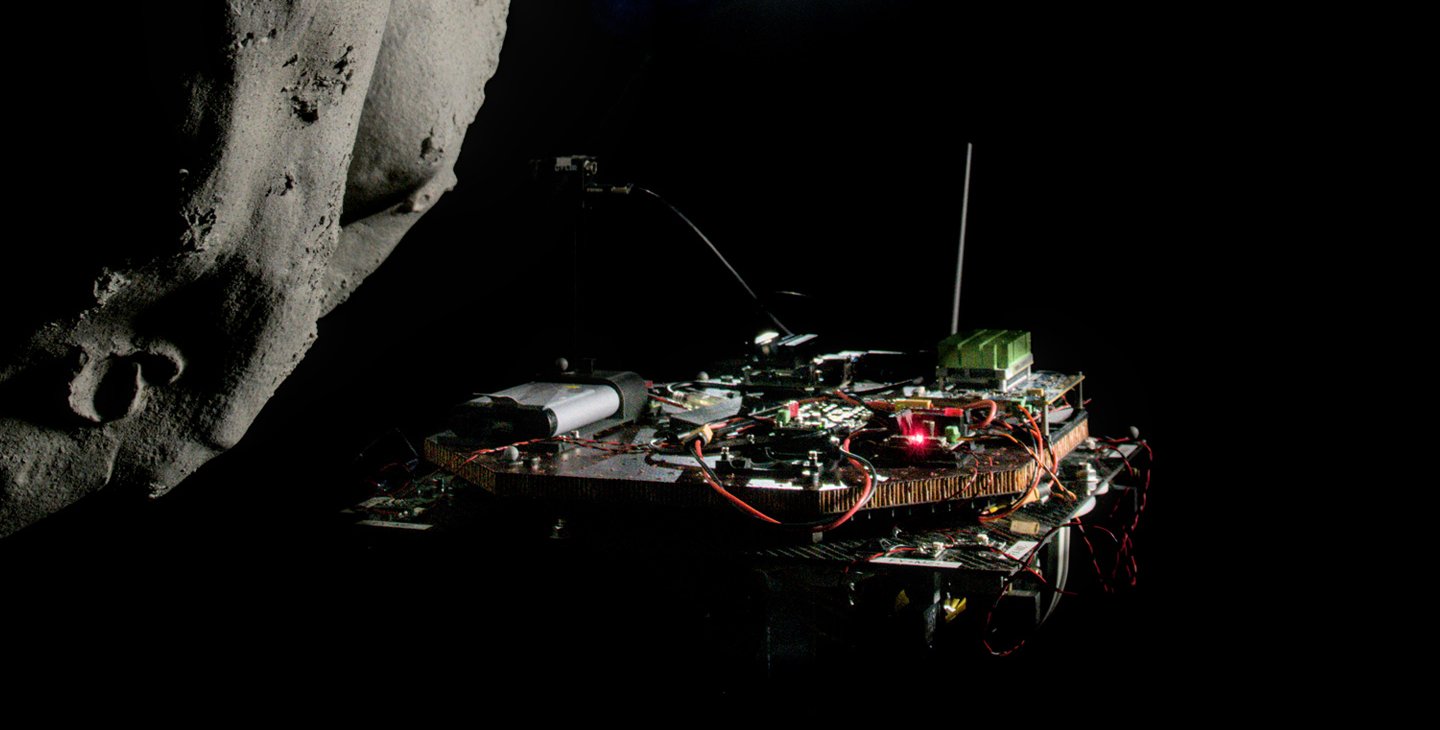
A New Approach to Autonomous Vehicle Safety
For humans throughout history, the sky has evoked thoughts of a vast emptiness, of a great vacant dome punctuated during the day by the sun, and at night by numerous tiny spots of light (and, periodically, by the moon). As we have ventured into space, both physically, with spacecraft, and optically, with a range of telescopic technologies, we now know that there is quite a lot of stuff up there.
This discovery has profound implications for the aerospace industry. Imagine, for example, a multibillion-dollar autonomous spacecraft that has been carefully designed and engineered for years is launched into space with precision calculations only to lose one of its thrusters and go sailing into an asteroid.
Historically, engineers have dealt with the possibility of equipment failure on board spacecraft in two main ways: First, by having a "safe mode" in which the spacecraft can do the least amount of damage to itself while scientists on the ground look at the data, make a diagnosis, and develop a solution; and second, by equipping autonomous vehicles with redundant systems. These allow a spacecraft to, for example, shut off a malfunctioning thruster and start using backup thrusters.
However, dangerous situations may crop up in space with little warning and insufficient time for space-to-ground communications. And though redundant systems have been quite effective, they add to the expense and heft of autonomous spacecraft.
This is why experiments are being conducted in the laboratory of Soon-Jo Chung, Bren Professor of Control and Dynamical Systems and senior research scientist at JPL, which Caltech manages for NASA, to streamline emergency features on autonomous vehicles such that they can diagnose and safely respond to encounters with other objects in real time. With new algorithms on board, spacecraft can test their own equipment and predict which future actions are most likely to keep them operating safely.
As one of the supervisors for this project, Fred Hadaegh, research professor in aerospace at Caltech and former JPL chief technologist, explains: "Having redundant systems is not always practical. It means the spacecraft has to be bigger, heavier, and more expensive than it would be otherwise. So, the idea here is that when a spacecraft encounters a problem, it can figure out what's not working and correct or adapt to that specific fault."
Chung's lab houses, among other things, an advanced multispacecraft dynamics simulator facility. "The simulator occupies a large room with a really flat floor," explains James Ragan, a PhD student in the Graduate Aerospace Laboratories of the California Institute of Technology (GALCIT) and lead author of this paper. "The model spacecraft uses air bearings so that it moves across the floor with near zero friction. At rest, it seems to be floating, and if you push it in one direction, it will keep going until it hits something, which is what space dynamics are like."
Ragan has programmed the robotic spacecraft simulator with what he and his co-authors call s-FEAST: Safe Fault Estimation via Active Sensing Tree Search. "Our s-FEAST algorithm rapidly 'dreams' about numerous possible futures that could result from actions it takes now," Ragan says. "Because the system is noisy, these futures are uncertain. There are multiple possible outcomes, which leads to a tree of possible branching futures. Each branch represents one possible way the future might happen, based on things the spacecraft controls—the test actions it selects—and also things it doesn't, such as observations coming from faulty sensors."
Chung adds: "What is innovative about our s-FEAST method is that we systematically solve the chicken and egg problem of estimating vehicle states, such as positions and velocities, and inferring failures or degradations, which are intrinsically coupled to one another."
When the spacecraft detects unexpected data, it turns to the s-FEAST algorithm, which runs test actions "similar to how you might carefully test your muscles when you feel an unexpected pain, and you want to figure out just what hurts and how to avoid actions that might further injure you," Ragan explains.
s-FEAST simultaneously spins out a range of possible futures and from those selects the course of action that appears most likely to diagnose what went wrong and also to avoid danger. In the case of this model, danger amounts to a collision course with an asteroid.
"The key idea here is that s-FEAST isn't replacing all spacecraft operations. It's your emergency response," Ragan says. "The spacecraft receives an internal signal that something is wrong, so s-FEAST takes over all the spacecraft's computing power to quickly assess what's going on and take remedial action. Once the danger is pinpointed and addressed, s-FEAST hands control back to the spacecraft's ordinary computing environment."
s-FEAST can also be used proactively. Say an autonomous spacecraft is about to take on a particularly risky or mission-critical action; s-FEAST can run a testing cycle to ensure that all systems are working properly before this action.
Chung and his co-authors envision that the proposed method will establish a new way of making expensive space exploration safer and more cost effective. "Space systems make autonomous operations necessary since we cannot grab and fix spacecraft and Mars helicopters operating in a world far away from us," Chung says. "Space is our ultimate ‘proving ground' for any autonomy research we do for Earth-based vehicle systems."
Not surprisingly, the s-FEAST algorithm that worked on the spacecraft simulator was adapted by the team to work on a ground track vehicle as well. Both experiments were successful, so s-FEAST technology holds great promise for autonomous vehicles on Earth as well as in space.
"Online Tree-based Planning for Active Spacecraft Fault Estimation and Collision Avoidance" is published in Science Robotics as an online feature article. A preliminary version of this paper received the 2023 American Institute of Aeronautics and Astronautics (AIAA) Guidance, Navigation, and Control Best Graduate Student Paper Award. Co-authors are Ragan, Caltech postdoc Benjamin Rivière (PhD '24), Hadaegh, and Chung. This work is funded by the Aerospace Corporation, JPL, the Defense Advanced Research Projects Agency Learning Introspective Control program, and the Technology Innovation Institute.
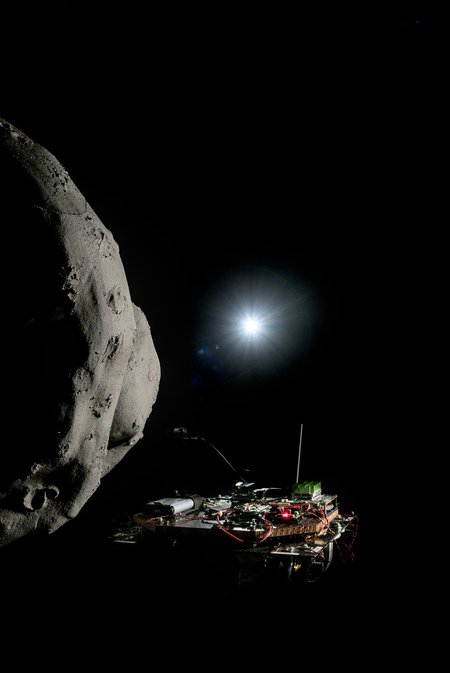