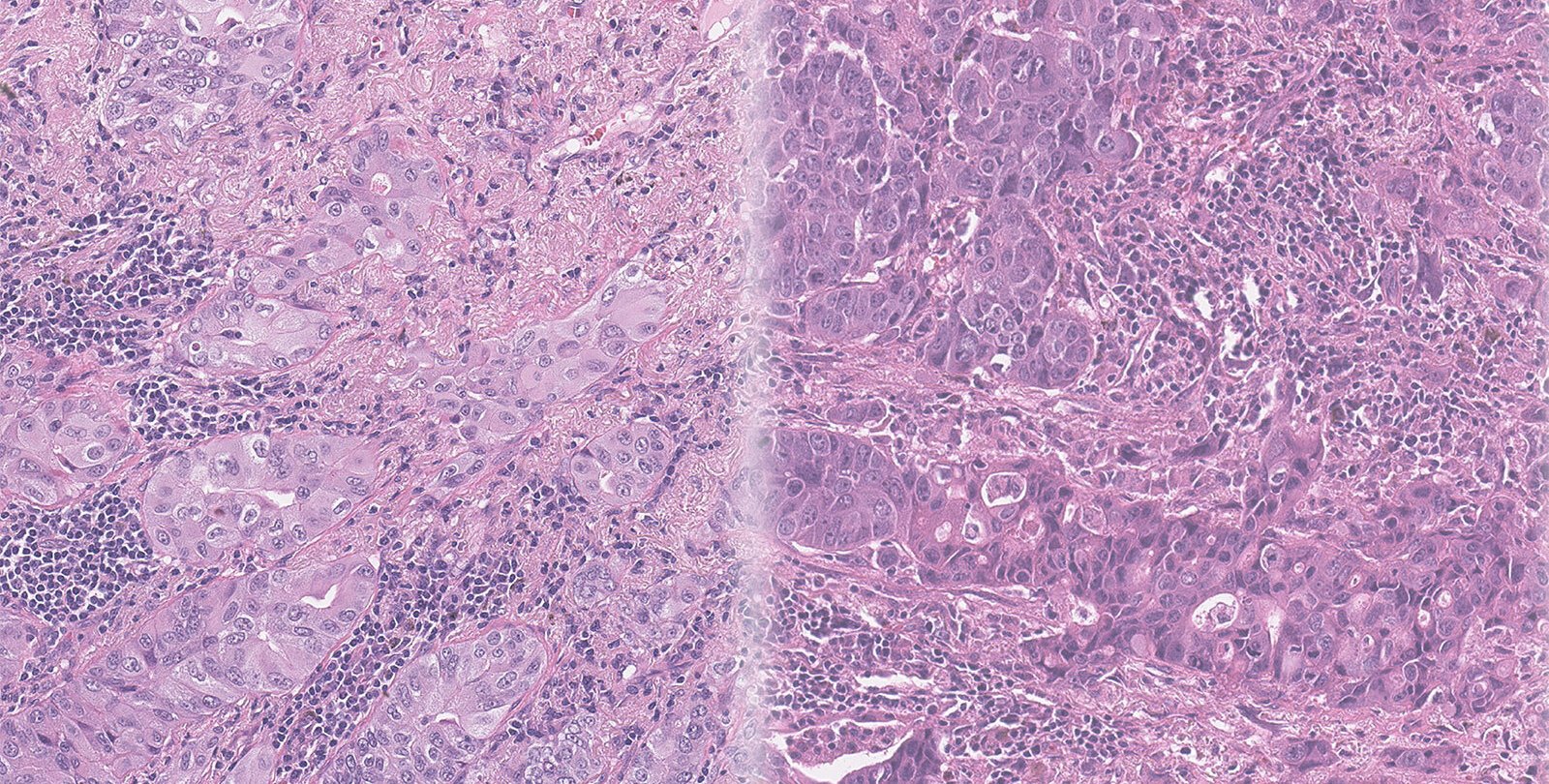
Using AI to Predict the Spread of Lung Cancer
For decades, scientists and pathologists have tried, without much success, to come up with a way to determine which individual lung cancer patients are at greatest risk of having their illness spread, or metastasize, to other parts of the body. Now a team of scientists from Caltech and Washington University School of Medicine in St. Louis has fed that problem to artificial intelligence (AI) algorithms, asking computers to predict which cancer cases are likely to metastasize. In a novel pilot study of non-small cell lung cancer (NSCLC) patients, AI outperformed expert pathologists in making such predictions.
These predictions about the progression of lung cancer have important implications in terms of an individual patient's life. Physicians treating early-stage NSCLC patients face the extremely difficult decision of whether to intervene with expensive, toxic treatments, such as chemotherapy or radiation, after a patient undergoes lung surgery. In some ways, this is the more cautious path because more than half of stage I–III NSCLC patients eventually experience metastasis to the brain. But that means many others do not. For those patients, such difficult treatments are wholly unnecessary.
In the new study, published this week in the Journal of Pathology, the collaborators show that AI holds promise as a tool that could one day aid physicians in this decision-making.
"Overtreatment of cancer patients is a big problem," says Changhuei Yang, the Thomas G. Myers Professor of Electrical Engineering, Bioengineering, and Medical Engineering at Caltech and an investigator with the Heritage Medical Research Institute. "Our pilot study indicates that AI may be very good at telling us in particular which patients are very unlikely to develop brain cancer metastasis."
Yang cautions that the work is only a first step and that a larger study is needed to validate the findings.
The team worked with data and biopsy images collected from 118 NSCLC patients at Washington University School of Medicine in St. Louis. Typically, a pathologist reviews such images, scouring them for abnormalities within the cells that might suggest the cancer is progressing.
Caltech electrical engineers led by Yang used hundreds of thousands of image tiles pulled from those 118 original biopsy images to train a type of AI program called a deep-learning network. They also provided follow-up data about which patients went on to develop brain metastases within five years of diagnosis and which did not.
"We essentially asked the network to learn from all these images, to pick out some features from the contextual information that could indicate something about a patient's outcome," says graduate student Haowen Zhou, first author of the new paper. Then the network was given 40 additional biopsy images and asked to determine whether the patients had gone on to experience brain metastases.
The AI network was able to correctly predict whether an individual NSCLC patient had experienced brain metastasis 87 percent of the time. In contrast, four expert pathologists who reviewed the same biopsy images were able to make the correct predictions only 57 percent of the time.
"Our study is an indication that AI methods may be able to make meaningful predictions that are specific and sensitive enough to impact patient management," says Richard Cote, head of the Department of Pathology & Immunology at Washington University School of Medicine and co-principal investigator of the new study. He notes that for the earliest-stage NSCLC patients (those classified as stage I), the AI results were even better than those for the whole study and that these predictions were based solely on basic, routinely processed microscopic slides. By giving the AI information on additional factors such as the severity of the disease and any additional biomarkers, the researchers expect that they will be able to improve the predictive powers of the AI program going forward.
Interestingly, the AI program does not indicate exactly what factors cause it to make certain predictions. So, the team is also working to uncover the subtle and complex features of tumor cells and their surroundings that the AI program might be homing in on.
"It's looking at what we would look at as a pathologist," Cote says. "But it's seeing more than we can see." Perhaps, he says, once scientists learn exactly what AI is focusing on, they will be able to develop new therapeutics to address those indicators.
Also looking forward, Yang's group at Caltech is interested in developing instrumentation and processes that would help scientists and clinicians collect more uniform and higher-quality biopsy images to boost the accuracy of AI predictions. "Once we can see what the AI is doing, we can start to think about how to design imaging and microscopy instruments to more optimally get the data that the AI wants," Yang says. "We can move away from imaging instruments designed for human use and move toward making instruments that are optimized for machine use."
Other Caltech co-authors on the paper, "AI-guided histopathology predicts brain metastasis in lung cancer patients," are graduate student Steven (Siyu) Lin and postdoctoral scholar research associate Simon Mahler. Additional co-authors from Washington University School of Medicine include Mark Watson, Cory Bernadt, Chieh-yu Lin, Jon Ritter, Alexander Wein, Sid Rawal, and Ramaswamy Govindan. The work was supported by the Heritage Medical Research Institute, Caltech's Center for Sensing to Intelligence, the Washington University in St. Louis School of Medicine Personalized Medicine Initiative, and the National Cancer Institute.